August 18, 2021
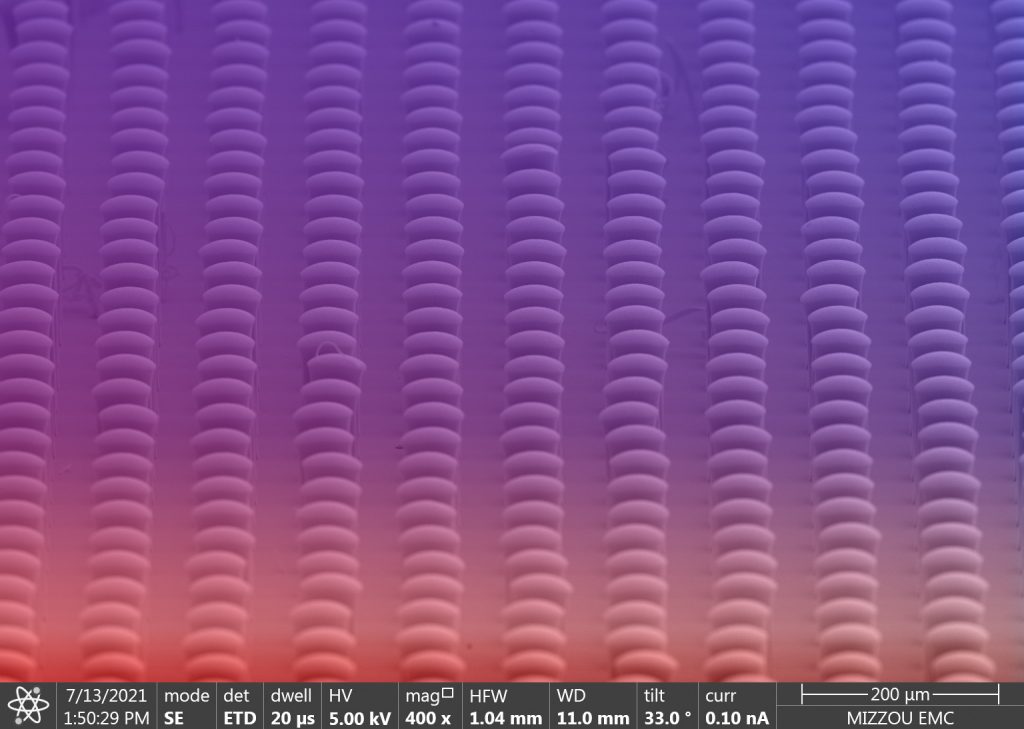
Carbon nanotubes have the potential to provide lightweight, flexible materials that could revolutionize the world. In their perfect form, these molecules — which are made of rolled sheets of carbon — are as rigid as diamond. They could be used to create automobile parts, construction materials, protective gear or durable electronic devices. Carbon nanotubes are so strong, in fact, Iron Man once replaced his metal shield with them to defeat a powerful villain.
But before humans can reap the benefits of these out-of-this-world molecules, researchers have to figure out how to mass produce them while making sure they maintain their superpowers.
That’s where Mizzou Engineering comes in. A research team has spent the past couple of years developing a simulation model to demonstrate how carbon nanotubes can be produced without losing their optimal properties.
The group has outlined their findings in npj Computational Materials, a publication of Nature partner journal.
Simulating carbon nanotube growth
Carbon nanotubes were discovered in the 1990s and are relatively easy to grow individually in a lab. However, when they grow collectively in a population, their collective interactions diminish their properties. While individual carbon nanotubes are as stiff as diamond, their properties are more rubber-like as an inter-connected population.
“During the synthesis process, a lot of complicated interactions between nanotubes happen, and the end result is that you typically do not obtain the great properties you wanted,” said Matt Maschmann, an associate professor in mechanical and aerospace engineering (MAE). “Many physical properties are substantially diminished, and you don’t get the engineering benefits you would expect when scaling from one nanotube,”
To get a glimpse of how carbon nanotubes could retain their best characteristics while growing in large groups, also known as carbon nanotube forests, Maschmann teamed up with researchers from electrical engineering and computer science (EECS) to simulate ideal conditions.
The group used machine learning algorithms to replicate that synthesis process and gain a better understanding of how the molecules interact. Within that simulation, the research team was able to alter parameters and test various growing conditions using physics.
“We use a physics-based simulation model to generate synthetic carbon nanotube forest images at nanometer resolution, and use these images to train a supervised AI classifier to predict one of 63 class types of carbon nanotube forests,” EECS Professor Kannappan Palaniappan said.
Rina Bao, a PhD student in computer science, worked with Palanippan and EECS Assistant Research Professor Filiz Bunyak Ersoy to develop a deep learning model called CNTNet that predicts CNT forest properties.
“In a second stage CNTNet also learns a regression model to predict physical properties such as carbon nanotube forest stiffness and buckling load with surprisingly high accuracy,” Palanippan said.
The goal is to grow carbon nanotubes by design, said Taher Hajilounezhad, a graduate student in MAE.
“For each specific application of CNTs, we need a different set of properties and a different structure,” he said. “In the semiconductor industry, we need smaller diameter and straighter CNTS, while for reinforcing composites we may need a thicker and denser CNT forest. Each application requires different process parameters to get the desired structure. This work allows us to predict those best synthesis conditions to get the desired set of properties from the beginning.”
While papers training AI systems relying completely on simulation models are rare, in this case, it was the only option. No one has been able to measure the growth of each CNT within a population in real time, so researchers have no “ground truth” by which to guide AI-based materials discovery.
“We prescribe the properties and attributes of every nanotube created in the synthetic model,” Maschmann said. “You wouldn’t know those things with physical experiments, and you certainly cannot control synthesis at that level.”
One goal is to create a catalog of the different models that can be further developed as more information is known, Associate Professor and Gillion Professor of Cyber Security Prasad Calyam said. Once additional properties and characteristics are discovered, the team can run models on top of their current simulation model with the ultimate goal of making a self-learning-based material discovery process.
“This paper is just the beginning,” Calyam said.
The next stage of the work is to image and characterize actual carbon nanotube forests. The group received a National Science Foundation grant last year for that work. Researchers will use a scanning electron microscope coupled with a synthesis system to watch carbon nanotube growth processes happening in real time, to gain a better understanding of when and why properties break down during collective growth.
While it generally takes about 20 years before a new material can be commercialized, Hajilounezhad said the physics-based simulation of CNT forest synthesis and characterization presents a reliable and powerful tool for training machine learning models.
“This approach will allow us to cut that time in half and it is surprisingly cost effective, because typically you have to do hundreds of expensive experiments to get to that point, but our simulation can get there with a fraction of the experiments,” he said. “The bigger picture is to help national security, human welfare and clean industries by using the significant beneficial engineering properties of nanomaterials.”
The work is an interdisciplinary collaboration funded, in part, by the National Science Foundation (Grants #1651538 and #2026847) and the Center for Cyber Education, Research and Infrastructure (Mizzou CERI Center), directed by Calyam. Last year, Bao won first prize in the poster competition at the College’s Research Day for his presentation, “Predicting Carbon Nanotube Forest Synthesis-Structure-Property Relationships Using Physics-based Simulation and Deep Learning.”