February 04, 2022
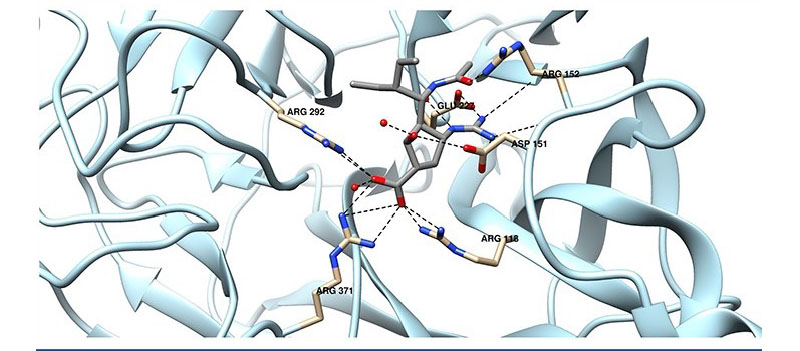
Prediction of protein-ligand interactions could speed up drug development.
It can take more than a decade and some $2.6 billion to get a new drug to market. And if COVID-19 has taught us anything, it’s that we can’t always wait that long for life-saving treatments. Now, a Mizzou Engineer has proposed a new deep learning system that would speed up drug development by more accurately predicting how drugs and proteins interact.
Jianlin “Jack” Cheng — William and Nancy Thompson Distinguished Professor in electrical engineering and computer science — outlined the system in a recent paper published by Oxford University Press’ Briefings in Bioinformatics. In the paper, he and his team examined existing computational approaches to predicting protein-ligand interactions and concluded that a more comprehensive system is needed.
Ligands are molecules such as drugs that bind to proteins and trigger a cellular response. In order to understand how a protein will respond to a ligand, researchers have to solve three problems. First, they must know how the molecule will bind to a target protein. In order for a drug to effectively block activity, it has to bind strongly to a specific protein such as the spike protein in the coronavirus. Secondly, they need to know where the ligand will bind within the protein. Finally, they need to know what 3D structure the protein and ligand will take once they become a complex, as that shape determines the cellular response.
“Current machine learning methods tend to treat the three problems as separate problems,” Cheng said. “But we think these three things are correlated, and we are proposing that they should be studied as a whole.”
Cheng’s research team is now working on developing such system. With a growing body of knowledge coming from existing computational systems and with a better understanding of how proteins develop into structures, it’s the ideal time to train highly sophisticated deep learning architectures to predict these protein-ligand interactions, he said.
“This will be an end-to-end system with everything automatically done by deep learning from beginning to end,” he said. “This is a great opportunity to develop a highly sophisticated deep learning method.”
Cheng was among the first to apply deep learning to predicting how proteins fold into specific structures, a problem that had vexed scientists for decades. In 2020, a Google-based company, DeepMind, largely solved the problem using an advanced end-to-end deep learning approach known as AlphaFold2.
“We can see how deep learning revolutionized the protein structure field when the protein prediction problem was largely solved,” Cheng said. “A similar revolution can happen in this vital field. That’s why we wanted to look at this from a fresh perspective and proposed some new ideas on where this field should go.”
The other co-authors of this paper include Mizzou graduate students Ashwin Dhakal and Cole McKay, and John J. Tanner, professor of chemistry and biochemistry.