April 28, 2022
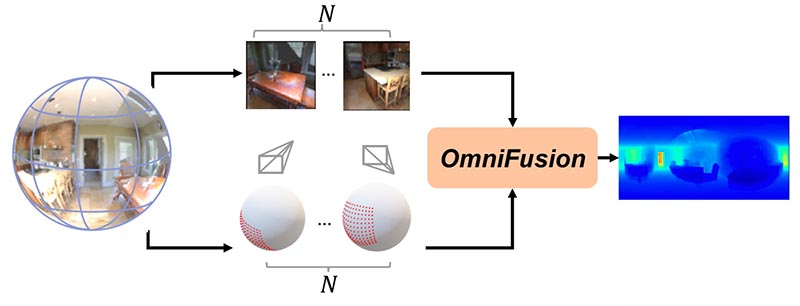
Omnifusion produces high-quality dense depth images (right) from a monocularimage (left) using a set of tangent images (N). The corresponding camera poses of the tangent images are shown in the middle row.
A Mizzou Engineering team has devised a new way to turn single panoramic images into 3D models. Researchers outlined the work in a paper that has been accepted to Computer Vision and Pattern Recognition (CVPR). Yuyan Li, a PhD candidate in computer science and lead author, will give an oral presentation at the conference — under the umbrella of IEEE’s Computer Society — in New Orleans this summer.
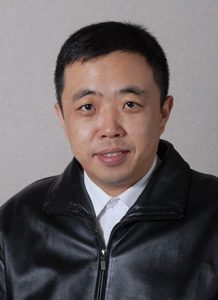
Ye Duan
“CVPR is one of the top conferences in AI (ranked #4 by Google scholar based on the h5-index among all scientific publications), so we’re fortunate to have a paper accepted,” said Ye Duan, associate professor of electrical engineering and computer science. “And getting accepted for an oral presentation is a big deal. I’m very happy for Yuyan.”
The research team developed a pipeline that estimates the depth of objects in a photo from the singular viewpoint of an ordinary camera, also known as a monocular perspective.
What’s novel about the system is its ability to capture geometric information. The pipeline, called OmniFusion, separates a 360-degree image — typically spherical — into a series of smaller images. Those images are used to train a machine to estimate the distance from the camera to pixels in the photo. The system then stitches the images back together — this time with correlating distances — allowing for creation a 3D environment with a more accurate field of depth.
Duan compared the work to being able to see with one eye closed. When you cover one eye, the distance of objects changes. However over time, your brain learns to understand how to perceive the world through that monocular view. Similarly, Duan and his students are training a machine to estimate and understand depth based on a single perspective.
There are numerous applications for the work, including developing more affordable ways to equip autonomous vehicles. Right now, some self-driving cars rely on light detection and ranging, or lidar, which uses laser beams to recreate a 3D representation of the vehicle’s surroundings. Lidar, however, is expensive.
The OmniFusion pipeline is based on a similar principle using more affordable cameras.
“Lasers measure the time of return. Since the speed of light is constant, you can measure the time of return and know the distance,” Duan said. “Now, we’re doing something similar using a single image and machine learning. We’re training a machine by providing images and corresponding depth information. By learning a lot of distances, the machine can begin to predict distance and translate it to depth.”
Experiments show that OmniFusion method greatly mitigates distortion, a well-known challenge when applying deep-learning methods to 360 images. It also achieves state-of-the-art performance against several benchmark datasets.
Be part of innovative research. Learn more about electrical engineering and computer science at Mizzou!