October 25, 2023
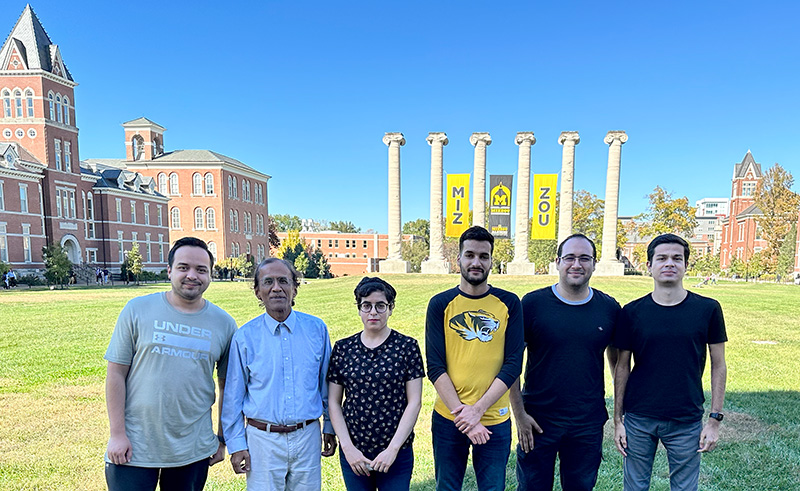
A Mizzou Engineering team took first and third place at a new competition that advanced methods to not only detect but also segment the 3D patterns of brain injury in newborns.
The BOston Neonatal Brain Injury Dataset for Hypoxic Ischemic Encephalopathy (BONBID-HIE) Lesion Segmentation Challenge was a Grand Challenge offered by the Medical Image Computing and Computer Assisted Intervention (MICCAI 2023) Society, and sponsored by leaders in the field from Boston Children’s Hospital and Harvard Medical School.
Hypoxic ischemic encephalopathy (HIE) is a brain injury that occurs in one to five out of every 1,000 babies. By 2 years of age, up to 60% of those affected will die or suffer permanent defects, said Imad Eddine Toubal, a Ph.D. student in computer science and member of the team.
The group — part of the Computational Imaging & Visualization Analysis (CIVA) Lab — also included Ph.D. students Elham Soltani Kazemi, Gani Rahmon, Taci Kucukpinar and Mohamed Almansour.
Teams were provided with a small dataset of magnetic resonance imaging (MRI) exams in infants with HIE lesions of varying shapes and distributions, as well as a few healthy infant brains. From there, participants were asked to characterize the 3D boundaries of the lesions to determine overall volume and affected brain regions.
To better identify the lesions from medical images, the group developed novel AI algorithms combining deep learning methods with traditional machine learning and image processing — a multi-pronged approach that separated Mizzou Engineers from the international competition.
Students had on their side Kannappan Palaniappan, a Curators’ Distinguished Professor of Electrical Engineering and Computer Science, as well as Mai-Lan Ho, Vice-Chair of Radiology at MU Health Care and an expert in advanced pediatric neuroimaging.
“Dr. Ho provided really good insight into the complexity of clinical imaging of newborns with HIE. She inspired the team not only to look at the challenge as a computer vision biomedical image analysis problem, but also brought in the clinical aspects and the profound benefits that timely diagnosis and intervention have on early childhood development,” Palaniappan said.
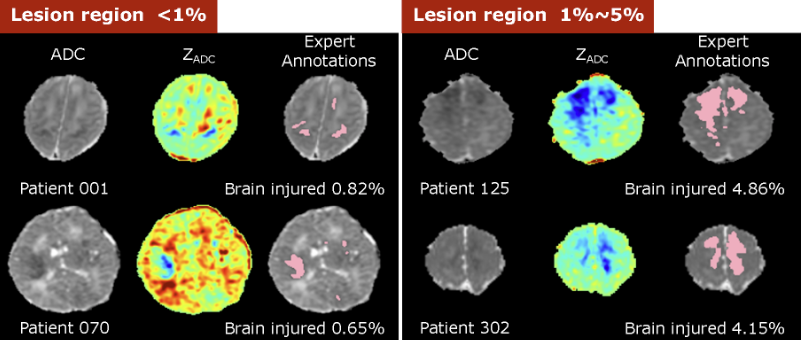
Students had to overcome several obstacles during the challenge. First, deep learning typically requires thousands of image volumes for training, particularly for 3D segmentation tasks—far greater than the 85 exams that were provided. Furthermore, traditional approaches to data augmentation, such as random flipping, cropping, and rotation, were not utilized since medical images must retain their normal anatomical relationships.
Additionally, the examinations were of heterogeneous quality, reflecting real-life data from the two different MRI scanners at Boston Children’s Hospital with different vendors and field strengths. Exams were also performed at varying postmenstrual ages and days after birth, such that the anatomy of the brains and character of the HIE lesions varied greatly. To make the algorithms more robust, Almansour resampled all image volumes using volume interpolation to achieve an optimal target voxel resolution for accurate segmentation.
Another problem unique to Mizzou was the fact that team members were sometimes separated by oceans. Almansour and Kucukpinar were at times out of the country during the duration of the challenge.
“It was definitely an international effort,” Kucukpinar said, who added that his Mizzou Engineering coursework prepared him well for the challenge. “It covered everything I’ve seen in classes.”
To solve the problem, team members focused on three different methodologies: a transformer network to look at images holistically; deep network loss functions to focus on key outcome metrics; and random forest machine learning combining deep and local features to provide more robust classification outputs.
Ultimately, their system performed over 10% better than baseline algorithms, matching the definition of ground truth—human expert annotations of HIE lesions—62% of the time.
Almansour said the challenge complemented work he’d done as part of his master’s program.
“It was nice working on a similar project, and Dr. Pal gave good ideas when things didn’t work as expected and we had suboptimal training data,” he said.
For Toubal, the workshop provided insights that will help him test algorithms he’s working on as part of his graduate research, including 3D segmentation of blood vessels and lymphatics in confocal microscopy volumes.
“It allowed me to test the algorithms I’ve been developing for my vessel segmentation research, see how adaptable they are to different datasets and identify shortcomings,” he said. “It also gave me a confidence boost seeing how well our algorithms performed in new areas and challenges.”
Dr. Ho praised the entire team for their determination and success.
“They were able to develop algorithms that surpassed other top computer science teams from across the world,” she said. “Very few groups could do the work they do, and it’s because of the vibrant interdisciplinary collaboration between engineering and medicine that happens at Mizzou.”
This was the second challenge Palaniappan’s research group has worked on this fall. Gani Rahmon, Elham Soltani and Imad Toubal, along with former Ph.D. student Noor Al-Shakani, also participated in the Visual Object Tracking and Segmentation (VOTS 2023) Benchmark, part of the 11-year-old VOT challenge that attracted 77 teams. Mizzou placed 16th overall and 5th in accuracy.
VOTS participants develop algorithms for identifying and tracking video objects in real time. This year, for the first time, students were asked to track multiple objects in both short-term and long-term videos. Teams developed algorithms that would find and track one or more specific objects, such as two boats in a larger fleet over the entire duration of a video, where the objects to be tracked are only “seen” or identified to the algorithm once in the first frame.
“I learned a couple of new deep learning architectures and large foundational models by participating in both challenges. These apply to the motion analysis research I’m working on and I am seeing promising results already,” Rahmon said.
Be part of innovative research. Learn more about the CIVA Lab.