July 09, 2023
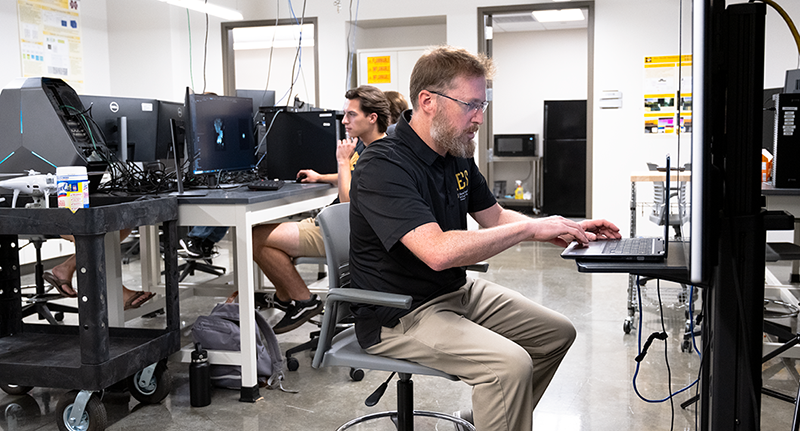
Generative artificial intelligence (AI) systems such as ChatGPT can provide a lot of convincing answers to user queries. What these models can’t do so well is explain how they derived their result and how confident they are in the output.
And large language models (LLMs) aren’t the only machines making decisions that impact us. Other types of neural networks are trained with images, not words. Thousands of photos are fed into systems until they can automatically recognize objects, such as tumors in medical images or traffic signals on the road. But they have limitations, too, because there simply aren’t enough images of the real world in its entirety to ensure, say, a self-driving car can recognize a stop sign in a new unknown environment when it’s obstructed by shadows or other contexts.
Derek Anderson, a professor in the Department of Electrical Engineering and Computer Science at Mizzou, has been studying these complex issues around AI for the past 20 years. His work in AI has spanned a variety of applications such as drones for humanitarian demining, drones in defense, geospatial analytics, material design. But at the root of it is engineering and understanding how AI algorithms process information and come to conclusion under uncertainty.
Anderson is committed to continuing that foundational research but admitted the launch of ChatGPT and sites like Google’s Bard or the New Bing from Microsoft have turned the field upside down.
“It’s the wild, wild west,” he said. “We’ve been almost entirely focused on methodologies to tackle domain specific tasks using datasets that allow AI to go deep. But this new generative era of AI has been given access to a much larger and diverse set of data, allowing it to go wide. Our field is evolving at an alarming rate. It’s exciting, scary and exhaustive.”
Beyond the explanation
Generative AI systems haven’t been built with the capacity to entirely explain why they provide the answers they give. This is why ChatGPT and other systems have made headlines for providing fictionalized legal cases and news sources that seem real but are entirely fabricated. Concerns in the academic domain have been raised with respect to copyright infringements and plagiarism.
AI ethics and law regulations are on their way to curtail issues like these, said Anderson, who sits on the Institute of Electrical and Electronics Engineers (IEEE) USA AI, IEEE vertical on societal implications of AI, and IEEE CIS Industry & Government (I&GA) committees.
One solution will be to ensure that future AI systems explain themselves. Anderson has created algorithms to do that, spitting out chains of numeric, text, and graphical explanations about the data and decision-making process.
But while Anderson and other computer scientists can make sense of those explanations, most of us can’t.
“While we can produce explanations for many aspects of AI, not all of these descriptions are relevant,” he said. “We need new ways to summarize and communicate useful information to people, or the public will not use or trust it.”
In a recent paper, published and presented at the IEEE Conference on AI, Brendan Alvey, a Ph.D. student in Anderson’s Mizzou INformation and Data FUsion Laboratory (MINDFUL), outlined a way to generate succinct natural language explanations of black box AI models, with associated uncertainty.
Alvey and Anderson can tailor their linguistic summarizations, which increases their usefulness across different types of users and criteria. This approach can be used to identify model weaknesses and data biases. For example, an AI model for computer vision on a drone that only works in a restricted set of flight conditions. The same principle applies when it comes to understanding model performance across human gender and race.
That’s where simulated data comes in.
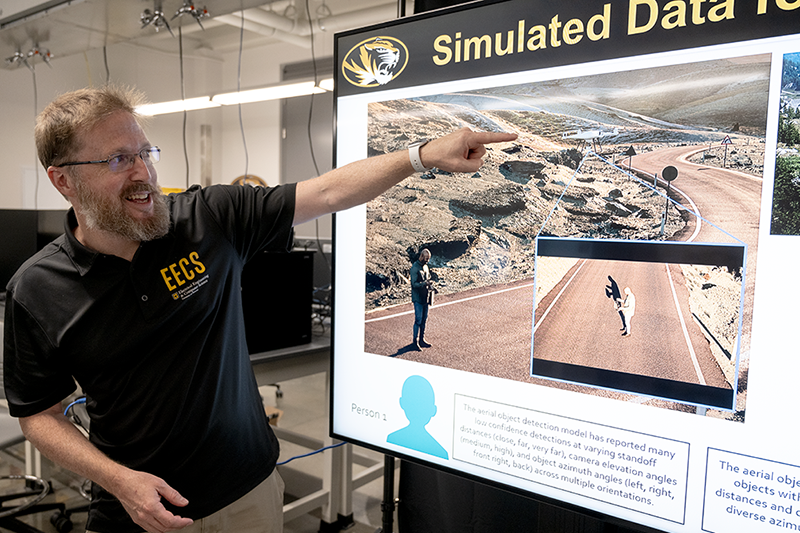
A virtual solution
One key component of Anderson’s research has been using simulated images rather than real-world photographs. This helps with respect to ground truth and increasing the size and variety of data to train an AI.
“Simulation is at the heart of everything we’re up to, from using AI for material development to autonomous drones and computer vision,” he said. “We are not alone. Large companies like Apple, NVIDIA, Microsoft and others have invested billions in producing and using simulated data.”
Anderson’s team primary uses the Unreal Engine, a suite of development tools that provides components to create virtual scenes and photorealistic visual spectrum imagery (aka “RGB images”). He also uses commercial plugins like Infinite Studio from Australia to simulate multispectral and infrared imagery. Tools like these allow researchers to not only increase the size of their datasets but also control range and variation. Scenes can be changed to account for different seasons or weather conditions, diverse skin tones and body sizes, and other scenarios like time and emplacement context.
Researchers can use a dataset entirely made up of these virtual scenes, comparing assets against real images to ensure they are accurate representations, or can use virtual images to complement and expand traditional datasets. Regardless, this will be vital to the future of AI technology.
“There’s not enough time or resources for us to collect every possible scenario (data) in the real world,” Anderson said. “Computing and (real) data gave rise to deep learning. Simulation could be our next leap. The hard question that we need to discover is what we need to be simulating for AI. It’s the consumer.”
Even as new text, image and video generators come online, Anderson is adamant that foundational concepts around AI must continue to be addressed before society can truly benefit from everything neural networks have to offer.
“We’ll learn to work alongside the tools that come along, but we also can’t get too focused on any specific one, because it might not be around six months from now,” he said. “The time between game-changing ideas in AI is constantly being reduced. However, explainable AI and simulation are persistent. We need them to innovate high quality, trustworthy and beneficial future AI.”
Explore the future of artificial intelligence, machine learning and neural networks at Mizzou Engineering. Apply today!