March 14, 2023
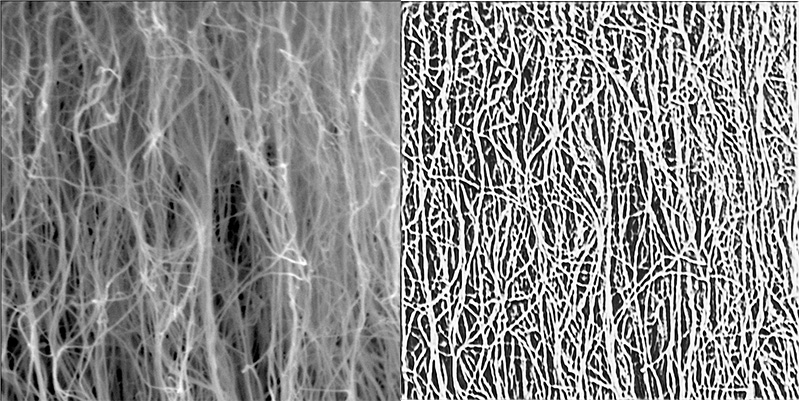
Mizzou Engineering researchers are another step closer to controlling the properties of carbon nanotubes growing in mass quantities.
Carbon nanotubes (CNTs) are nanoscale cylindrical carbon molecules that have unique electric, mechanical and thermal properties. CNTs are flexible, lightweight and strong material that could have a variety of real-world applications.
While optimal CNT properties are readily observed for an isolated CNT at the nanoscale, properties are significantly degraded when they are synthesized en masse at the microscale. Simultaneously growing CNT populations, known as CNT forests, interact and entangle in ways that are not fully understood with the net effect of modulating their collective properties.
Before engineers can solve that problem, they must first be able to measure and characterize how individual CNTs are assembled within forests.
In their latest paper, Matt Maschmann, Filiz Bunyak and co-authors have outlined a deep learning technique to segment carbon nanotube forests in scanning electron microscopy (SEM) images. This technique allows them to detect individual CNTs within a forest which can help eventually characterize their properties.
“This technique allows us to quantifiably interpret SEM images of complex and interacting CNT forest structures,” said Maschmann, who is an associate professor of mechanical and aerospace engineering and co-director of the MU Materials Science & Engineering Institute. “My dream for this would be to have an app or plug-in where anyone looking at an SEM image of carbon nanotubes could see histograms representing the distributions of CNT diameters, growth rates and even estimated properties. There’s a lot of work to do to make that happen, but it would be phenomenal. And I think it’s realistic.”
The novelty of the team’s technique is that it’s self-supervised. That’s important because manually tracing hundreds of individual nanotubes within a complex CNT forest image to train a machine learning model would be too time consuming and labor intensive. Furthermore, because nanotubes are so small and because they intertwine and clump together, human annotations would likely vary, resulting in inaccurate training data.
Bunyak’s PhD student Nguyen P. Nguyen developed the self-guided model to convert raw images into line drawings allowing the model to identify CNT strands and determine their orientation more clearly.
“No previous paper has segmented CNTs at this level.” said Bunyak, an assistant professor of electrical engineering and computer science. “But the innovation goes beyond this. The proposed approach can be used for analysis of other curvilinear structures such as biological fibers or synthetic fibers.”
The simulation code is focused on three parameters: diameter, density and growth rate. But there are other phenomena happening within CNT forests, too, that researchers want to understand, said Kannappan Palaniappan, Curators’ Distinguished Professor of Electrical Engineering and Computer Science and co-author.
“For example, the angle at which they grow is a variable as they can topple over or get tangled,” he said. “How do you characterize those values? We need to isolate individual CNTs and see what they do both individually and how they interact. There’s no chance of us doing that without this kind of technique.”
“Self-supervised Orientation-Guided Deep Network for Segmentation of Carbon Nanotubes in SEM Imagery” was presented at the European Conference on Computer Vision in February. Co-authors include Nguyen P. Nguyen, a PhD student in computer science, Ramakrishna Surya, a PhD student in mechanical and aerospace engineering, and Prasad Calyam, Greg L. Gilliom Professor of Cyber Security.
This study is part of the team’s ongoing investigation around carbon nanotubes.