May 22, 2023
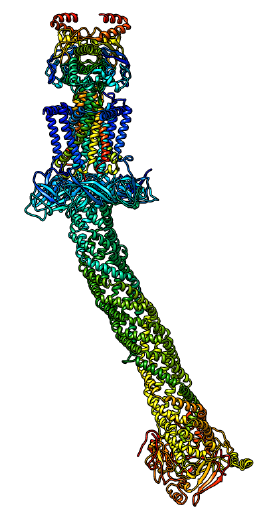
A Mizzou Engineer has received funding from the National Science Foundation to develop a tool that will predict how a protein functions based on its order of amino acids.
Jianlin “Jack” Cheng envisions developing open source software that would allow a user to enter the sequence, then the system would predict not only how that string of amino acids will form into a structure but also the role it will carry out within a cell. Additionally, the system would pinpoint the specific site of the protein that carries out the function.
Because proteins are the building blocks of life, applications span from engineering drought-resistant crops to advanced drug development.
“This will allow researchers to understand what kind of molecular function the protein has,” said Cheng, Thompson Professor of Electrical Engineering and Computer Science. “For instance, if a protein is promoting tumor growth in a cancer patient, scientists could design a drug to prohibit the site of that activity and slow or stop it from growing.”
Cheng is using a deep transformer model, a large language model with some similarity to the one that powers ChatGPT, the popular generative artificial intelligence (AI) program that generates text based on user prompts. Like words, protein sequence is the language of biological systems.
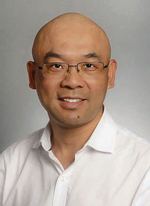
The team is developing three types of deep transformer models. A one-dimensional sequence-based transformer considers the sequence of amino acids. A 2D graph transformer considers how proteins interact with one another, analyzing what these interactions will do. And a 3D-equivariant graph transformer takes into consideration the protein structure and different sites within the protein that carry out specific tasks.
This is the latest milestone in Cheng’s impressive career around protein prediction. In 2012, he and his students were the first to demonstrate the superiority of deep learning for predicting protein structure in the 10th Critical Assessment of Techniques for Protein Structure Prediction (CASP10). In 2020 CASP14 experiment, Google-owned Deep Mind unveiled an advanced deep learning method AlphaFold2 that predicted protein structure with unprecedented accuracy. In 2022 CASP15 experiment, the Cheng Group further improved the accuracy of AlphaFold2-based protein structure prediction by 8-10%.
“We’re leveraging cutting-edge protein structure methods and using AlphaFold2 in this particular project,” Cheng said. “The language model methodology is rather new in this domain. This is an interesting area, and we’re putting a lot of research effort into it. We’re pretty excited about this work.”
Read more about Cheng’s work in the field of protein prediction.