June 01, 2023
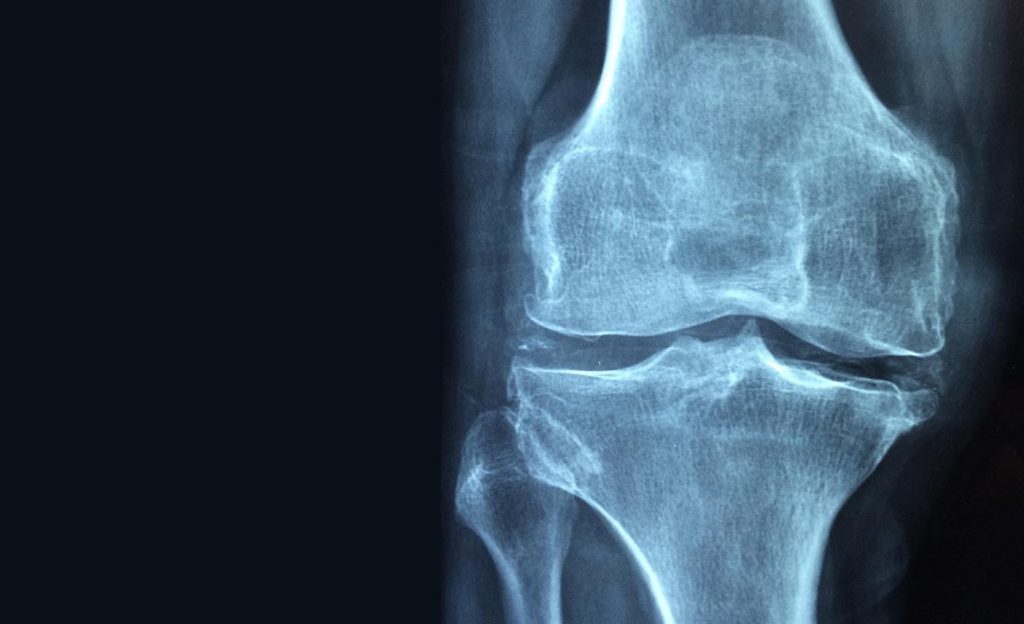
Osteoarthritis is the most common type of arthritis, impacting more than 32 million adults in the U.S. It’s a degenerative disease that makes it hard to move, and current assessment techniques require needles to extract fluid from joints.
Now, an interdisciplinary research team at Mizzou has demonstrated a way to use non-invasive electromyography, or EMG, signals to assess lower body movements in osteoarthritis patients. The work is promising for patients, as it could lead to improved personalized care and rehabilitation.
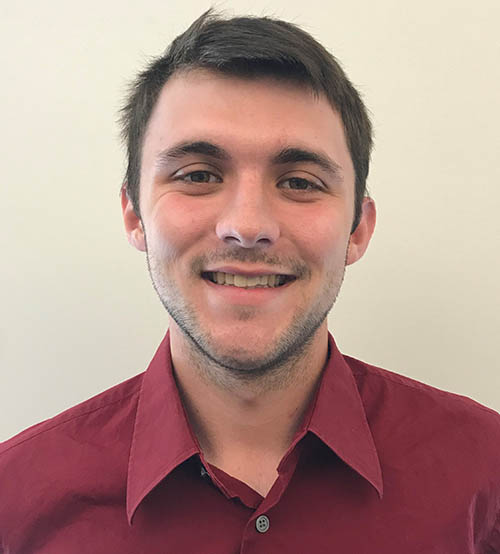
“Osteoarthritis hurts, so patients don’t want to move. But they need to move more to keep their joints loose,” said Richard Byfield, a Ph.D. candidate in mechanical engineering and first author of the paper published in Biomedical Engineering Advances. “This is a preliminary study, but the impact could lead to EMG signals being captured in real time to help patients move their joints in a wider range of motion and could help inform decisions around prevention, treatment and rehabilitation.”
Byfield and Associate Professor Jian Lin worked with Matt Guess, BS ME ’21, along with Guess’s father, Trent Guess, an associate professor in the Department of Physical Therapy and Orthopaedic Surgery.
For the study, they measured lower body movement of 24 patients, specifically looking at joint angles and ground reaction force as the patients stepped onto and off of a box.
The team captured joint angles and ground reaction force for a variety of extensions and movements in the knees, hip, pelvis and ankles. They used that data to train a machine learning algorithm to correlate the measurements with EMG signals.
What’s novel about the work is that it takes into account all lower body movements, analyzing 20 degrees of motion, while other studies have looked at three degrees of motion for a single joint or one degree of motion for multiple joints.
“It was pretty accurate, with a 3-4% relative error,” Byfield said. “This means a machine learning algorithm can predict joint angles and ground reaction force based on EMG signals alone. Those signals can predict a range of motion.”
They also found that five principal components within the data were able to explain at least 80 percent of variation. By requiring fewer inputs in future models, researchers can decrease computational demand.
The team used a machine learning algorithm known as an echo state network, a form of recurrent neural networking that uses a reservoir computing process to find hidden correlations. This is especially important when analyzing biological data, which tends to be noisy and chaotic.
Byfield is defending his Ph.D. dissertation this coming year but hopes to further develop this research in the future.
“I’d like to enter academia and have a joint appointment with biomedical engineering to build a rehabilitation system,” he said. “There are a lot of potential applications, not only for osteoarthritis but also other musculoskeletal conditions and movement disorders. I’m excited about the possibilities.”
Engineer solutions to real-world problems. Apply to Mizzou Engineering today!