September 28, 2023
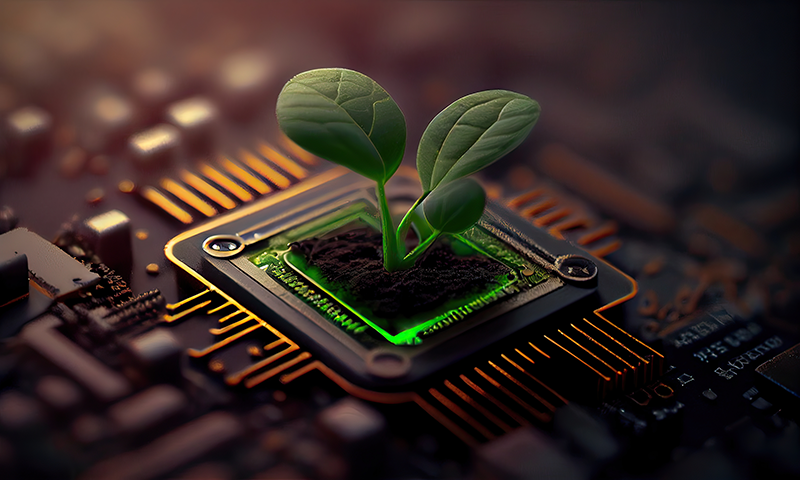
A Mizzou Engineering team is devising a method to make computer chips designed to run deep neural networks (DNNs) not only reliable, but also energy efficient and sustainable.
Khaza Anuarul Hoque recently received collaborative funding as the Lead Principal Investigator from the National Science Foundation (NSF)’s Design for Environmental Sustainability in Computing (DESC) program for the work. Hoque is an assistant professor of electrical engineering and computer science and is director of the Dependable Cyber-Physical Systems (DCPS) Lab.
“Deep neural networks are everywhere,” Hoque said. “They’re used in everything from autonomous cars and computers to health care and aerospace applications. But these are power-hungry models, making them impractical for energy-constrained devices.”
Hardware approximation methods can help in reducing the power consumption in these chips with a slight accuracy loss, and these techniques are gaining lot of attention. However, Hoque and his team have shown that such approximation can also make these chips three times more vulnerable to permanent manufacturing faults.
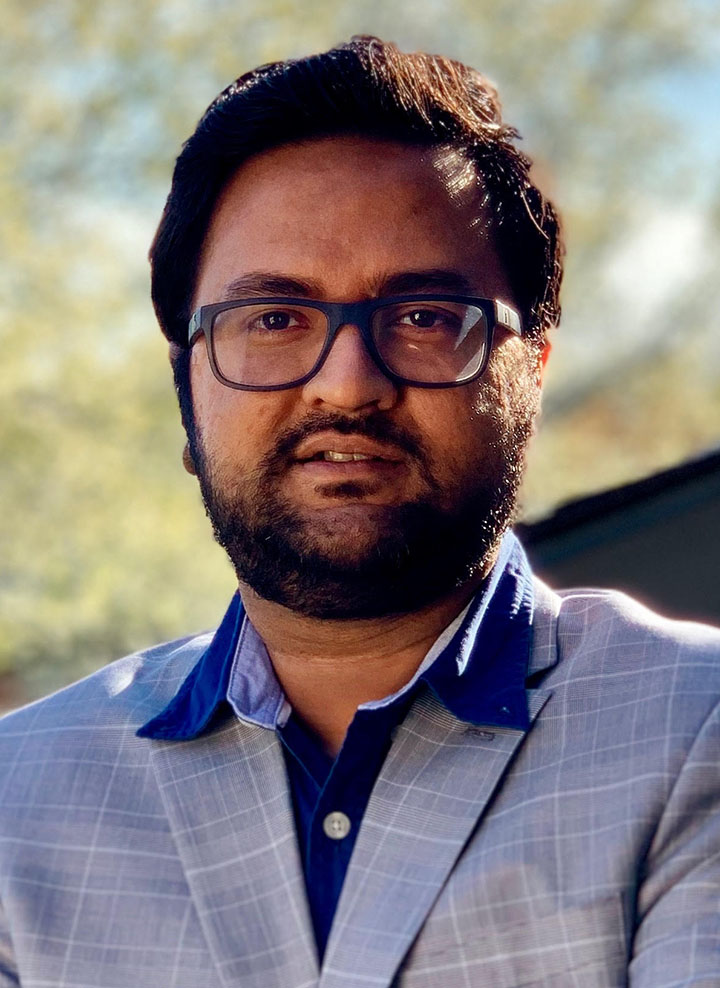
“A significant number of chips may need to be discarded if they are faulty. These faults can be due to manufacturing issues or simply due to the chips aging. This means, with the increasing use of these AI chips, we will see a dramatical increase of e-waste which is a great threat to our environment,” Hoque said. “We want these chips to not only be energy efficient but also sustainable by prolonging their overall lifetime.”
He’s doing this by coming up with techniques to detect and correct faults or bypass them.
The project has three objectives. First, the team is examining techniques focused on creating approximate DNNs that are dependable and sustainable using new approaches to finding optimal neural network architectures. Second, they’re investigating methods to deal with faults that arise after the creation and deployment of the chips by either repairing or bypassing faulty components or retraining the network to address faults. Finally, they’re creating a tool to show how well approximate DNNs perform when compared to other metrics.
“Ultimately, we want to fix these chips by novel fault mitigation techniques and reuse them so we don’t need to throw faulty chips away,” he said. “We’re optimizing for performance, energy efficiency and sustainability.”
The work is expected lead to new tools and codes that will be available to the broader DNN and cyber-physical systems communities through open-source software. Additionally, outcomes will be used to develop new curriculum and laboratory exercises for computer and electrical engineering students.
Hoque will lead this 3-year project and will also collaborate with Benjamin Carrion Schaefer, the PI at the University of Texas at Dallas site.
Interested in developing theories, methods and tools to support cyber-physical systems? Learn more about the DCPS Lab at Mizzou Engineering!