January 02, 2023
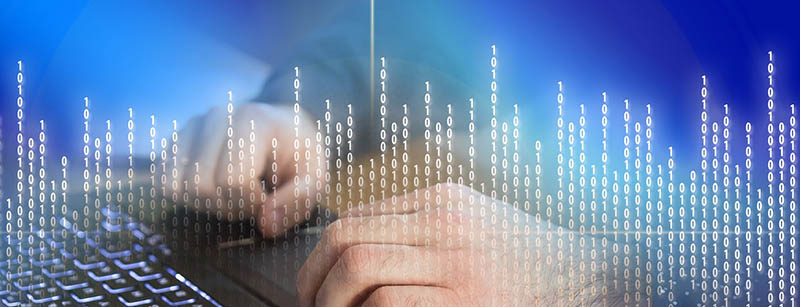
A Mizzou Engineering research team led by Assistant Professor Khaza Anuarul Hoque will present two papers at the Design Automation and Test in Europe Conference and Exhibition (DATE), an A1-rated conference, in the area of hardware and electronic design automation (EDA), in Belgium this coming spring.
Both papers further investigation around spiking neural networks, a type of machine learning that recognizes objects based on binary code — the “0” and “1” system used in digital computation.
“Machine learning hardware is very power hungry,” said Hoque, director of the Dependable Cyber-Physical Systems (DCPS) Laboratory. “One reason spiking neural networks are gaining in popularity is that they are both brain inspired and energy efficient compared other deep neural networks.”
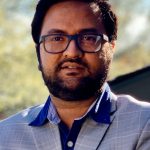
PhD student Ayesha Siddique will present her paper on improving the reliability of SNNs used in hardware. PhD student Syed Tihaam Ahmad will present on ensuring those systems are resilient against cyber threats.
“The process of presenting my ideas and work is exciting,” said Ahmad, who will be presenting at a conference for the first time. “And I’ll get to meet with different research groups.”
Siddique presented at DATE’s virtual conference last year and is looking forward to meeting academics and industry leaders in person this year.
“And they will be meeting some of the brightest minds in the field,” Hoque said. “All of the top researchers will be there, so presenting and getting feedback from them will be a great experience for the students and our research.”
SNN Reliability
Spiking neural networks lend themselves well to hardware because they provide the benefits of training a machine to recognize and classify objects and do so in a way that’s faster and more efficient than other methods.
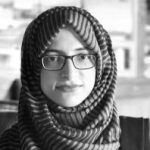
However, manufacturing faults are common during the chip manufacturing process. Those flawed chips produce inaccurate results, so in most cases they’re thrown away.
Siddique helped develop a technique that retrains the chip, so it is able to identify and bypass faulty components.
“Even if there is a small percentage of fault in hardware, the accuracy drops a lot,” she said. “Our mitigation technique recovers the accuracy close to the perfect chip, and it increases the reliability of the hardware.”
SNN Resiliency
While spiking neural networks are already energy efficient, using approximation techniques reduces energy requirements even more at the cost of slight accuracy loss. However, doing so increases a systems susceptibility to malicious activity.
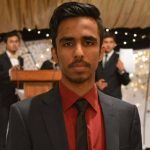
In his paper, Ahmad proposed a design methodology to provide a more secure version of SNNs using two novel defense methods: precision scaling and approximate quantization-aware filtering. Both techniques significantly improved the robustness of approximate SNNs.
“We found the sweet spot for these ultra-low-power spiking neural networks to make them more resilient toward different kinds of cyber-attacks,” Ahmod said.
Siddique was a co-author of his paper.
The three will attend the DATE conference April 17-19, 2023, in Antwerp, Belgium.
Be part of internationally recognized research. Apply to Mizzou Engineering today!