October 13, 2021
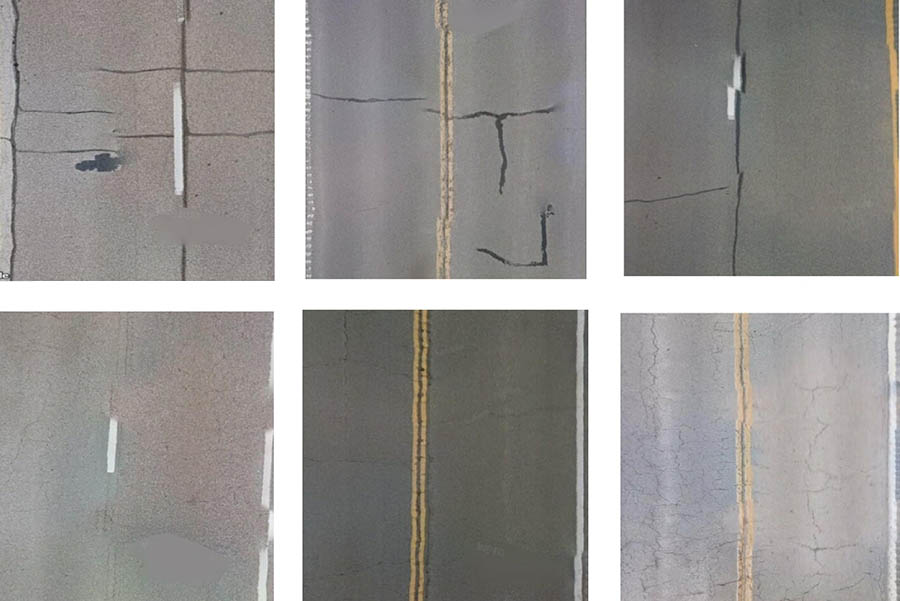
Researchers are using artificial intelligence to classify various types of pavement cracks, such as these examples from Missouri roadways.
Traffic engineers could have a smarter way of identifying asphalt problems and prioritizing pavement projects, thanks to cutting-edge research from Mizzou Engineering. The team is training a machine to automatically classify types of cracks and potholes on city streets in Kansas City.
There are multiple types of types of pavement cracks, all of which can cause further problems or indicate more serious issues. Historically, city employees have had to rely on expensive equipment or manually view and identify these cracks — which isn’t feasible for all 6,000 lane miles the city of Kansas City maintains.
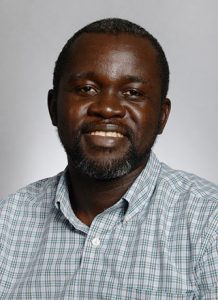
Adu-Gyamfi
“The goal is to use machine learning to do that,” said Yaw Adu-Gyamfi, an assistant professor in the Civil and Environmental Engineering (CEE) Department. “We’re currently in the process of training students to know the types of distresses so they can teach the machine learning model how to do it.”
The group is imputing that data into the computer model based on images of surface distresses from the city, Google Street images and other sources. Additionally, researchers have developed a mobile app that city workers can mount on vehicle dashboards to record videos of the roadways they travel.
While pavement can visibly exhibit dozens of types of deteriorations, some of those distresses can indicate underlying issues, said Bill Buttlar, the Glen Barton Chair in Flexible Pavement Technology in CEE. In Kansas City, for instance, a surface crack could reveal foundational problems on roads that are more than 100 years old.
“Each one of the distresses we identify on the surface can give us clues about subsurface deficiencies,” said Buttlar, who is also director of the Missouri Center for Transportation Innovation. “Sometimes we get clues that the problem is not with the new surfacing we just installed but a deeper foundational problem. That tells city engineers that they may want to schedule an area of pavement for deeper repair or total reconstruction.”
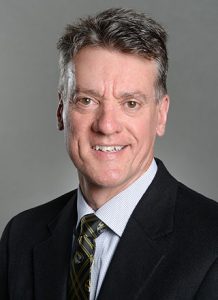
Buttlar
The work should ultimately help Kansas City engineers more efficiently maintain the roadways and prioritize repairs.
Buttlar also plans to work with Kansas City to use recycled materials to repair roadways. Similar to work being done on Stadium Boulevard in Columbia, he envisions creating new types of asphalt with recycled pavement, old tires and plastic wastes.“There are only so many hours in the day for humans to evaluate road data because they also have to work on other projects and infrastructure systems,” Buttlar said. “The machine will allow us to go through all the data — every square inch of roadway — and aggregate the findings so humans can make high-level decisions based on the microlevel information.”
“Some of the things we’re doing on Stadium Boulevard have never been tried in Missouri, and we would like to apply what we learn and the best practices to the city of Kansas City,” he said. “They have access to recycled materials and could make great use of those in city pavement.”
The team expects Kansas City to have tools they can implement within a year. Then, Buttlar and Adu-Gyamfi say both aspects of the project could be replicated to benefit cities throughout the U.S.
“The materials we’re looking at are new, and the machine learning techniques are new,” Buttlar said. “Missouri is one of the only states leading the way in this research and development.”