March 26, 2021
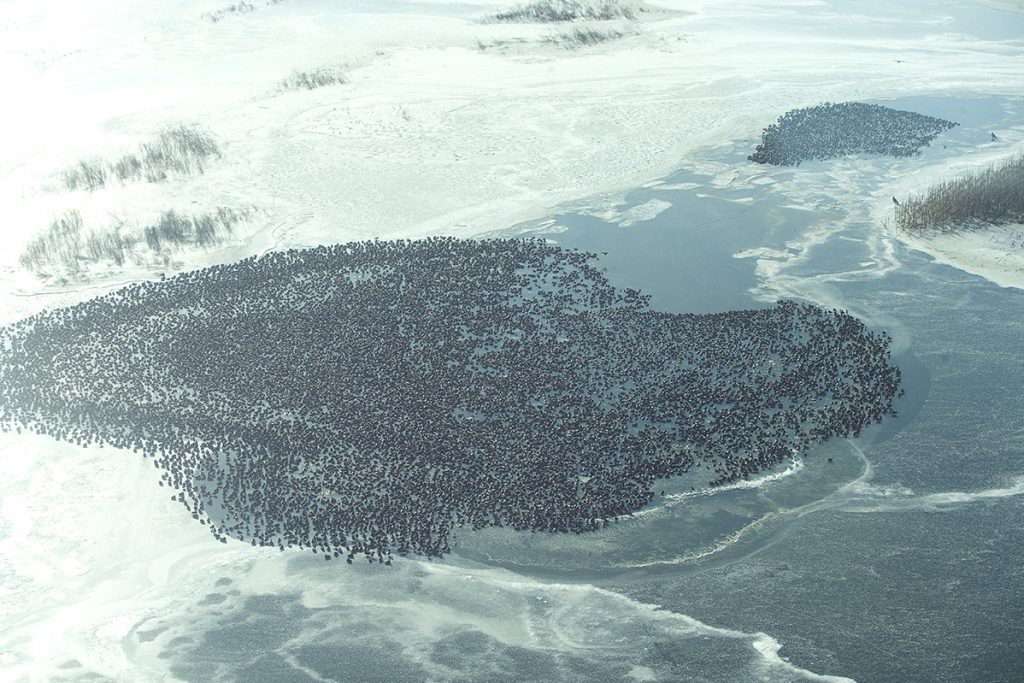
Professor Yi Shang and team are developing a deep learning system that will better identify small objects such as waterfowl in images.
Artificial Intelligence (AI) is good at recognizing a single bird in an image. Where it falls short is when it tries to identify hundreds of tiny birds in an aerial photo. Professor Yi Shang and his research team have been working for three years to see where AI can improve its vision when it comes to small objects.
“Computer vision has made big breakthroughs in recent years because of deep learning,” said Shang, who is also director of graduate studies in the Electrical Engineering and Computer Science Department. “Facial recognition is considered solved — computers recognize faces better than humans do. But if it’s a small face in a crowd, or a small object in an image, low resolution and lack of object details make recognition more difficult.”
Specifically, Shang’s lab is developing several deep learning methods that can better identify birds and regions of invasive species from aerial images taken by drones. Last year, he received a grant from the Missouri Department of Conservation (MDC) to develop a system to use drones and deep learning to automatically count waterfowl populations and survey invasive species.
To do so, students are manually identifying and labeling each bird within an image to train AI what to look for even when birds are partially hidden. It takes hours to label an image with hundreds of birds, but the work is necessary to improve the deep learning model’s ability to detect small objects.
The goal is to reduce the need to do manual counting in the future.
“For the easy-to-see birds, we can count them with confidence, but there will always be some we’re not confident about,” said Nickolas Wergeles, an assistant professor of professional practice in computer science and information technology. “So there may always have to be some human intervention to double check. But this will still save them a lot of work even if it’s not 100% perfect.”
The research team is also working with MDC to develop a system that can identify invasive plants, such as reed canary grass. This will be a more difficult project: Researchers will have to identify these invasive species, which grow alongside other types of plants. One solution is to collect images in early spring or fall in hopes that reed canary grass green sooner or brown later in the season.
Shang also plans to use a multispectral camera that may reveal some useful light signatures between the different species to better distinguish them.
“The computer won’t know the targets until being trained on examples,” Shang said. “Humans may not be able to detect the difference based on visible light. The multispectral camera may provide information not visible to human eyes.”
Shang, Wergeles and recent PhD graduates Yang Li and Peng Sun recently experimented with existing state-of-the-art deep learning methods on small object detection to test their performance using the waterfowl images and other public benchmark datasets. They outlined challenges with each method and identified possible solutions in a survey paper published in the international journal Expert Systems and Applications.
“When you use existing machine learning models, they usually don’t perform well out of the box,” Shang said. “There are a number of parameters that need to be fine-tuned to achieve good result. Recognizing birds, for instance, is different from recognizing faces, so you have to adapt and retrain the models.”