October 28, 2021
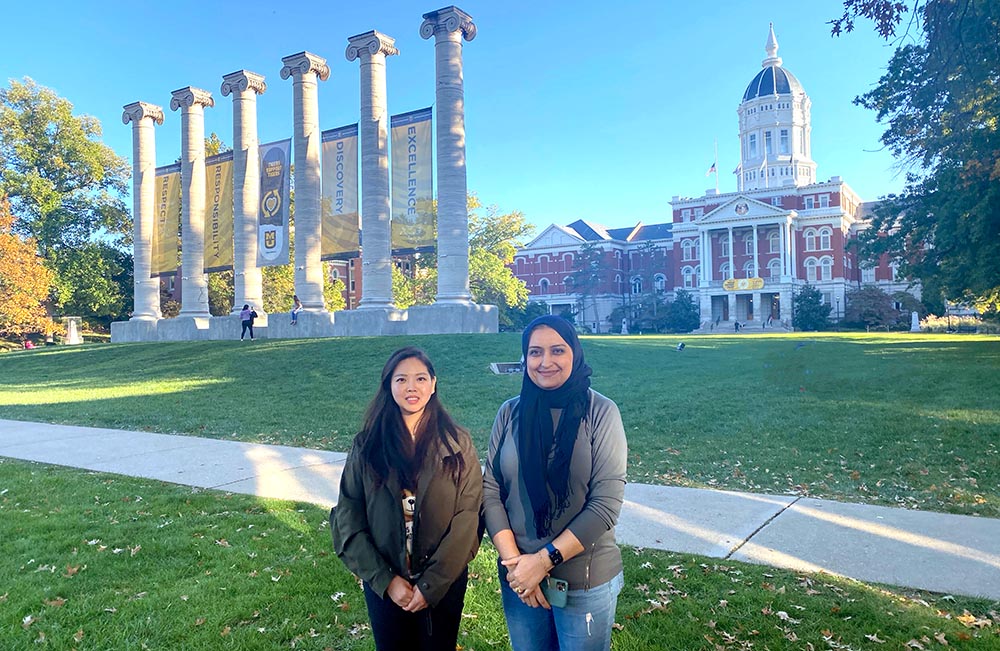
Rina Bao and Noor Al-Shakarji developed a system to segment and track cells from microscopy video images.
A Mizzou Engineering team won a Best Paper Award at an international workshop earlier this month. Rina Bao, who just completed her PhD, and PhD student Noor Al-Shakarji received the accolade for developing a two-stream deep learning network to identify, segment and track cells from microscopy video images. Theirs was one of two papers recognized at the Computer Vision for Automated Medical Diagnosis workshop, part of the International Conference on Computer Vision. The workshop was organized by researchers from academia, government and industry including Stanford, Johns Hopkins, Vanderbilt, ShanghaiTech, NIH, and Google Brain.
Being able to identify and monitor cells in microscopy images has numerous benefits for clinical diagnosis applications and biomedical research, said Kannappan Palaniappan, professor of electrical engineering and computer science, the senior author and advisor for the group.
“It’s the foundation for early drug discovery and understanding biomedical mechanisms for how cells communicate with each other and how they sense their environment,” he said. “A lot of life science research starts at the cellular level, so understanding cell dynamics in a quantitative way is very powerful. But it has been difficult to achieve in the past because of the large variety of cell types, high cell densities and their complex behaviors.”
In the past, cell segmentation and tracking has been done manually, which is expensive and time consuming, Bao said. It’s also subjective, as the person labeling the cells might select a subset of cells that are easier to track or cells that are visible for a longer period of time biasing the parameter estimate.
There are existing methods to automatically detect cells by identifying their boundaries. However, these methods cannot handle difficult cases where cells overlap or deform, altering the boundary contours, Bao said.
She and Al-Shakarji developed a pipeline to use a shape marker-based approach to detect, separate and track cells. Instead of focusing only on boundaries, Bao’s DMNet deep learning approach generates guided markers that assists the segmentation module to split cells that are overlapping or touching.
Once cells are detected and accurately segmented, Al-Shakarji’s M2-Tracker-Lite model follows individual cells over time, including keeping track of cell divisions and lineage. The approach scales to many hundreds of cells.
The team tested the dual-stream marker guided deep network on 13 different cell types from the Cell Tracking Challenge (CTC-6) benchmark, including HeLa cells, which are used in cancer research, and mesenchymal stem cells, which can differentiate into fat, muscle, bone, cartilage and other cell types. The DMNet plus M2Track pipeline had the best overall rank performance on the 2D cell videos outperforming three of the most competitive methods from Karlsruhe Institute of Technology, Germany, Purdue University and California Institute of Technology.
“It is a testament to the strength of our ongoing collaborative research in microscopy imaging with Mizzou Bond Life Sciences Center investigators that we received the best paper award since much of the emphasis of the workshop was on clinical image analysis for computer-aided diagnosis,” Palaniappan said.
The project was the first biomedical data set Bao and Al-Shakarji have worked with, although they’ve used similar detection and tracking approaches in the past for other research in Palaniappan’s Computational Imaging & VisAnalysis Lab (CIVA). Bao has focused on remote sensing algorithm design and deep learning including building detection and segmentation, while Al-Shakarji has focused on single and multi-object tracking for surveillance, aerial motion imagery and video detection of moving objects.
“It shows the versatility of the lab’s research in being able to cut across domains from biomedical to remote sensing research,” Palaniappan said. “Both Rina and Noor are brilliant at adapting tools and techniques across domains.”
Filiz Bunyak, assistant research professor in electrical engineering and computer science and team mentor, agreed, noting that the team didn’t have much time to work with the biomedical data set.
“Rina and Noor have developed robust tools, which is the reason they were able to be so successful,” she said. “Everything they’d done until that point was very powerful and robust, and they were able to adapt what they had built for other domains for the cell tracking application.”
Bao and Al-Shakarji both recommend Mizzou Engineering for graduate school, praising the opportunities they’ve had in the CIVA lab.
For Bao, it’s been life changing. Prior to her PhD, she hadn’t worked on medical applications. This week, she started as a post-doctoral fellow in a joint position between Harvard Medical School and Boston Children’s Hospital.
“Working with Dr. Pal in graduate school for my PhD research on deep learning architectures has helped me in shaping my future career,” she said. “I definitely recommend it. I love Mizzou.”
The research was partially funded by awards from the National Institutes of Health NIND R01-NS110915 and Army Research Laboratory W911NF-18-20285.
The paper DMNet: Dual-Stream Marker Guided Deep Network for Dense Cell Segmentation and Lineage Tracking can be found online at CVF open access.